Abstract
Human activities are altering the existing patterns of Land Use Land Cover (LULC) and Land Surface Temperature (LST) on a global scale. However, long-term trends of LULC and LST are largely unknown in many remote mountain areas such as the Karakorum. . The objective of our study therefore was to evaluate the historical changes in land use and land cover (LULC) in an alpine environment located in Islamabad Capital Territory, Pakistan. We used Landsat satellite pictures (namely Landsat 5 TM and Landsat 8 OLI) from the years 1988, 2002, and 2016 and applied the Maximum Likelihood Classification (MLC) approach to categorize land use classes. Land Surface Temperatures (LST) were calculated using the thermal bands (6, 10, and 11) of Landsat series data. The correlation between the Human Modification Index (HMI) and LULC as well as LST was evaluated by utilizing data from Google Earth Engine (GEE). Over the study period, the urbanized area increased by 9.94%, whilst the agricultural and bare soil areas decreased by 3.81% and 3.94%, respectively. The findings revealed a significant change in the LULC with a decrease of 1.99% in vegetation. The highest LST class exhibited a progressive trend, with an increase from 12.27% to 48.48%. Based on the LST analysis, the built-up area shows the highest temperature, followed by the barren, agricultural, and vegetation categories. Similarly, the HMI for different LST categories indicates that higher LST categories have higher levels of human alteration compared to lower LST categories, with a strong correlation (R-value = 0.61) between HMI and LST. The findings can be utilized to promote sustainable urban management and for biodiversity conservation efforts. The work also has the potential of utilizing it to protect delicate ecosystems from human interference and to formulate strategies and regulations for sustainable urban growth, including aspects of land utilization and zoning, reduction of urban heat stress, and urban infrastructure.
Keywords:
land use land cover; land surface temperature; global human modification; maximum likelihood classification; urban heat island
Resumo
As atividades humanas estão alterando os padrões existentes de uso e cobertura do solo (LULC) e temperatura da superfície terrestre (TST) em escala global. No entanto, as tendências a longo prazo de LULC e LST são, em grande parte, desconhecidas em muitas áreas montanhosas remotas, como em Karakorum. O objetivo do nosso estudo foi, portanto, avaliar as mudanças históricas no uso e cobertura da terra (LULC) em um ambiente alpino localizado no Território da Capital Islamabad, Paquistão. Utilizamos imagens de satélite Landsat (nomeadamente Landsat 5 TM e Landsat 8 OLI) dos anos de 1988, 2002 e 2016 e aplicamos a abordagem de Classificação de Máxima Verossimilhança (MLC) para categorizar as classes de uso da terra. As temperaturas da superfície terrestre (TST) foram calculadas usando as bandas térmicas (6, 10 e 11) dos dados da série Landsat. A correlação entre o Índice de Modificação Humana (HMI) e o LULC, bem como o LST, foi avaliada utilizando dados do Google Earth Engine (GEE). Durante o período de estudo, a área urbanizada aumentou 9,94%, enquanto as áreas agrícolas e de solo descoberto diminuíram 3,81% e 3,94%, respectivamente. Os resultados revelaram uma mudança significativa no LULC, com uma diminuição de 1,99% na vegetação. A classe LST mais alta apresentou tendência progressiva, com aumento de 12,27% para 48,48%. Com base na análise do LST, a área construída apresentou a temperatura mais elevada, seguida pelas categorias árida, agrícola e vegetação. Da mesma forma, o HMI para diferentes categorias de LST indicou que as categorias mais altas de LST apresentaram níveis mais elevados de alteração humana em comparação com as categorias mais baixas de LST, com uma forte correlação (valor R = 0,61) entre HMI e LST. As conclusões podem ser tomadas como base para promover a gestão urbana sustentável e para esforços de conservação da biodiversidade. O trabalho também tem o potencial de ser utilizado para proteger ecossistemas delicados da interferência humana e para formular estratégias e regulamentos para o crescimento urbano sustentável, incluindo aspectos de utilização e zoneamento da terra, redução do estresse térmico urbano e infraestrutura urbana.
Palavras-chave:
uso e cobertura do solo; temperatura da superfície terrestre; modificação humana global; classificação de máxima verossimilhança; ilha de calor urbano
1. Introduction
Anthropogenic activities, such as increasing agricultural productivity, expanding settlements, developing transportation networks, and exploiting resources, continuously alter land surfaces. (Rahimi, 2019RAHIMI, L., 2019 [viewed 20 January 2023]. The relationship between the use of land cover and urban thermal islands using Landsat 8: case study of Sanandaj. Journal of Radar and Optical Remote Sensing [online], vol. 2, no. 4, pp. 79-92. Available from: https://sanad.iau.ir/journal/jrors/article_669740.html
https://sanad.iau.ir/journal/jrors/artic...
; Das and Angadi, 2020DAS, S. and ANGADI, D.P., 2020. Land use-land cover (LULC) transformation and its relation with land surface temperature changes: a case study of Barrackpore Subdivision, West Bengal, India. Remote Sensing Applications: Society and Environment, vol. 19, pp. 100322. http://doi.org/10.1016/j.rsase.2020.100322.
http://doi.org/10.1016/j.rsase.2020.1003...
). The alterations of natural land surfaces made by humans have adverse environmental effects, such as the reduction of biodiversity, flora, and temperature changes. Urban regions in both developed and developing countries have seen significant negative impacts due to increased human activities (Willie et al., 2019WILLIE, Y.A., PILLAY, R., ZHOU, L. and ORIMOLOYE, I.R., 2019. Orimoloye, I.R. Monitoring spatial pattern of land surface thermal characteristics and urban growth: a case study of King Williams using remote sensing and GIS. Earth Science Informatics, vol. 12, no. 4, pp. 447-464. http://doi.org/10.1007/s12145-019-00391-2.
http://doi.org/10.1007/s12145-019-00391-...
; Ali et al., 2014ALI, K., AHMAD, H., KHAN, N. and JURY, S., 2014. Future of Abies pindrow in Swat District, Northern Pakistan. Journal of Forestry Research, vol. 25, no. 1, pp. 211-214. http://doi.org/10.1007/s11676-014-0446-1.
http://doi.org/10.1007/s11676-014-0446-1...
; Herbeck et al., 2011HERBECK, L.S., UNGER, D., KRUMME, U., LIU, S.M. and JENNERJAHN, T.C., 2011. Typhoon-induced precipitation impact on nutrient and suspended matter dynamics of a tropical estuary affected by human activities in Hainan, China. Estuarine, Coastal and Shelf Science, vol. 93, no. 4, pp. 375-388. http://doi.org/10.1016/j.ecss.2011.05.004.
http://doi.org/10.1016/j.ecss.2011.05.00...
). In the same manner, the increasing growth of the human population and migration patterns have led to the expansion of urban areas, especially in developing nations. This development has given rise to a range of health and environmental concerns, such as heatstroke, asthma, cardiovascular ailments, the Urban Heat Island (UHI) effect, and pollution (Vaidyanathan et al., 2020VAIDYANATHAN, A., MALILAY, J., SCHRAMM, P. and SAHA, S., 2020. MMWR - heat-related deaths: United States, 2004-2018. MMWR. Morbidity and Mortality Weekly Report, vol. 69, no. 24, pp. 729-734. http://doi.org/10.15585/mmwr.mm6924a1. PMid:32555133.
http://doi.org/10.15585/mmwr.mm6924a1...
; Sahani et al., 2022SAHANI, J., KUMAR, P., DEBELE, S. and EMMANUEL, R., 2022. Heat risk of mortality in two different regions of the United Kingdom. Sustainable Cities and Society, vol. 80, pp. 103758. http://doi.org/10.1016/j.scs.2022.103758.
http://doi.org/10.1016/j.scs.2022.103758...
).
UHI refers to a metropolitan or city region that experiences higher temperatures compared to the surrounding rural areas as a result of human activities. UHI is influenced by several factors, such as the release of heat caused by human activities, the modification of land use and land cover, and the presence of air pollution (Yamamoto, 2005YAMAMOTO, Y., 2005. Measures to mitigate urban heat islands. Quartely Review, vol. 18, pp. 65-83.; Nuruzzaman, 2015NURUZZAMAN, M., 2015. Urban heat island: causes, effects and mitigation measures-a review. International Journal of Environmental Monitoring and Analysis, vol. 3, no. 2, pp. 67-73. http://doi.org/10.11648/j.ijema.20150302.15.
http://doi.org/10.11648/j.ijema.20150302...
). Land cover pertains to the extent of natural features on the land, whereas land use refers to how much land is utilized by humans. However, these two aspects are frequently examined in conjunction due to their strong interdependence (Verburg and Overmars, 2009VERBURG, P.H. and OVERMARS, K.P., 2009. Combining top-down and bottom-up dynamics in land use modeling: exploring the future of abandoned farmlands in Europe with the Dyna-CLUE model. Landscape Ecology, vol. 24, no. 9, pp. 1167-1181. http://doi.org/10.1007/s10980-009-9355-7.
http://doi.org/10.1007/s10980-009-9355-7...
; Buyantuyev and Wu, 2010BUYANTUYEV, A. and WU, J., 2010. Urban heat islands and landscape heterogeneity: linking spatiotemporal variations in surface temperatures to land-cover and socioeconomic patterns. Landscape Ecology, vol. 25, no. 1, pp. 17-33. http://doi.org/10.1007/s10980-009-9402-4.
http://doi.org/10.1007/s10980-009-9402-4...
; Hu et al., 2021HU, S., FU, Z., JACKSON SAMUEL, R.D. and ANANDHAN, P., 2021. Application of active remote sensing in confirmation rights and identification of mortgage supply-demand subjects of rural land in Guangdong Province. European Journal of Remote Sensing, vol. 54, no. 2, pp. 396-404. http://doi.org/10.1080/22797254.2020.1790996.
http://doi.org/10.1080/22797254.2020.179...
). Urban regions undergo land use and land cover (LULC) changes that convert natural vegetation and agricultural land into impermeable surfaces (Buyantuyev and Wu, 2010BUYANTUYEV, A. and WU, J., 2010. Urban heat islands and landscape heterogeneity: linking spatiotemporal variations in surface temperatures to land-cover and socioeconomic patterns. Landscape Ecology, vol. 25, no. 1, pp. 17-33. http://doi.org/10.1007/s10980-009-9402-4.
http://doi.org/10.1007/s10980-009-9402-4...
). The Normalized Difference Vegetation Index (NDVI) is commonly applied to examine the relationship between LST and vegetation cover (Guha et al., 2018GUHA, S., GOVIL, H., DEY, A. and GILL, N., 2018. Analytical study of land surface temperature with NDVI and NDBI using Landsat 8 OLI and TIRS data in Florence and Naples city, Italy. European Journal of Remote Sensing, vol. 51, no. 1, pp. 667-678. http://doi.org/10.1080/22797254.2018.1474494.
http://doi.org/10.1080/22797254.2018.147...
; Weng et al., 2004WENG, Q., LU, D. and SCHUBRING, J., 2004. Estimation of land surface temperature–vegetation abundance relationship for urban heat island studies. Remote Sensing of Environment, vol. 89, no. 4, pp. 467-483. http://doi.org/10.1016/j.rse.2003.11.005.
http://doi.org/10.1016/j.rse.2003.11.005...
; Ullah et al., 2023ULLAH, W., AHMAD, K., ULLAH, S., TAHIR, A.A., JAVED, M.F., NAZIR, A., NAZIR, A., ABBASI, A.M., AZIZ, M. and MOHAMED, A., 2023. Analysis of the relationship among land surface temperature (LST), land use land cover (LULC), and normalized difference vegetation index (NDVI) with topographic elements in the lower Himalayan region. Heliyon, vol. 9, no. 2, e13322. http://doi.org/10.1016/j.heliyon.2023.e13322. PMid:36825192.
http://doi.org/10.1016/j.heliyon.2023.e1...
).
The effects of LULC changes on land surface temperature (LST) have been extensively investigated. (Connors et al., 2013CONNORS, J.P., GALLETTI, C.S. and CHOW, W.T.L., 2013. Landscape configuration and urban heat island effects: assessing the relationship between landscape characteristics and land surface temperature in Phoenix, Arizona. Landscape Ecology, vol. 28, no. 2, pp. 271-283. http://doi.org/10.1007/s10980-012-9833-1.
http://doi.org/10.1007/s10980-012-9833-1...
; Zhang et al., 2016ZHANG, Y., BALZTER, H., LIU, B. and CHEN, Y., 2016. Analyzing the impacts of urbanization and seasonal variation on land surface temperature based on subpixel fractional covers using Landsat images. IEEE Journal of Selected Topics in Applied Earth Observations and Remote Sensing, vol. 10, no. 4, pp. 1344-1356. http://doi.org/10.1109/JSTARS.2016.2608390.
http://doi.org/10.1109/JSTARS.2016.26083...
; Fu and Weng, 2016FU, P. and WENG, Q.A., 2016. time series analysis of urbanization induced land use and land cover change and its impact on land surface temperature with Landsat imagery. Remote Sensing of Environment, vol. 175, pp. 205-214. http://doi.org/10.1016/j.rse.2015.12.040.
http://doi.org/10.1016/j.rse.2015.12.040...
; Das et al., 2022DAS, T., JANA, A., MANDAL, B. and SUTRADHAR, A., 2022. Spatio-temporal pattern of land use and land cover and its effects on land surface temperature using remote sensing and GIS techniques: a case study of Bhubaneswar city, Eastern India (1991-2021). GeoJournal, vol. 87, suppl. 4, pp. 765-795. http://doi.org/10.1007/s10708-021-10541-z.
http://doi.org/10.1007/s10708-021-10541-...
). Zhou and Wang (2011)ZHOU, X. and WANG, Y.C., 2011. Dynamics of land surface temperature in response to land‐use/cover change. Geographical Research, vol. 49, no. 1, pp. 23-36. http://doi.org/10.1111/j.1745-5871.2010.00686.x.
http://doi.org/10.1111/j.1745-5871.2010....
determined that alterations in LST are predominantly associated with modifications in impermeable surfaces. Studies have focused on the effects of human-induced changes in LULC on fractional vegetation coverage and surface roughness. For example, Fu (2003)FU, C., 2003. Potential impacts of human-induced land cover change on East Asia monsoon. Global and Planetary Change, vol. 37, no. 4, pp. 219-229. http://doi.org/10.1016/S0921-8181(02)00207-2.
http://doi.org/10.1016/S0921-8181(02)002...
explored how changes in LULC impact LST in the context of urbanization in the metropolitan area of Atlanta, USA. The relationship between LST and landscape characteristics, as well as socio-ecological and socioeconomic variables, was evaluated in multiple Chinese cities (Huang et al., 2011HUANG, G., ZHOU, W. and CADENASSO, M.L., 2011. Is everyone hot in the city? Spatial pattern of land surface temperatures, land cover and neighborhood socioeconomic characteristics in Baltimore, MD. Journal of Environmental Management, vol. 92, no. 7, pp. 1753-1759. http://doi.org/10.1016/j.jenvman.2011.02.006. PMid:21371807.
http://doi.org/10.1016/j.jenvman.2011.02...
). Some researchers conducted a study on the relationship between LST and vegetation cover and built-up area at a regional scale. They discovered that changes in LULC have a major impact on LST and the creation of UHI (Jenerette et al., 2007JENERETTE, G.D., HARLAN, S.L., BRAZEL, A., JONES, N., LARSEN, L. and STEFANOV, W.L., 2007. Regional relationships between surface temperature, vegetation, and human settlement in a rapidly urbanizing ecosystem. Landscape Ecology, vol. 22, no. 3, pp. 353-365. http://doi.org/10.1007/s10980-006-9032-z.
http://doi.org/10.1007/s10980-006-9032-z...
). The developments in remote sensing technology offer a great tool for accurately assessing the amount of land use and land cover changes and their influence on LST. Satellite-based remote sensing is used to collect spatial data, which is then converted into useful information for estimating and understanding changes in land use and LST. A GIS-based computer system is used to collect, store, analyze, and display this spatial information (Weng, 2001WENG, Q.A., 2001. A remote sensing? GIS evaluation of urban expansion and its impact on surface temperature in the Zhujiang Delta, China. International Journal of Remote Sensing, vol. 22, no. 10, pp. 1999-2014.; Ghosh et al., 2022GHOSH, S., KUMAR, D. and KUMARI, R., 2022. Assessing spatiotemporal dynamics of land surface temperature and satellite-derived indices for new town development and suburbanization planning. Urban Governance, vol. 2, no. 1, pp. 144-156. http://doi.org/10.1016/j.ugj.2022.05.001.
http://doi.org/10.1016/j.ugj.2022.05.001...
). The combination of remote sensing (RS) and geographic information systems (GIS) yields accurate and thorough data regarding the spatial distribution of LST and LULC alterations. Remote sensing data have been established as a crucial resource for change detection due to their frequent updates, digital format that is suitable for computation, comprehensive view, and a broader range of options for geographical and spectral resolutions (Xiuwan, 2002XIUWAN, C., 2002. Using remote sensing and GIS to analyse land cover change and its impacts on regional sustainable development. International Journal of Remote Sensing, vol. 23, no. 1, pp. 107-124. http://doi.org/10.1080/01431160010007051.
http://doi.org/10.1080/01431160010007051...
; Coops et al., 2006COOPS, N.C., WULDER, M.A. and WHITE, J.C., 2006. Identifying and describing forest disturbance and spatial pattern. In: M.A. WULDER and S.E. FRANKLIN, eds. Understanding forest disturbance and spatial pattern. Boca Raton: CRC Press, pp. 31-61.).
Previous studies have demonstrated that the impact of human activities on urban thermal dynamics cannot be comprehensively grasped solely through parameters like land use and land cover (LULC) changes, as these parameters fail to account for the cumulative effects of human activities (Kar and Liou, 2019KAR, S.K. and LIOU, Y.A., 2019. Influence of land use and land cover change on the formation of local lightning. Remote Sensing, vol. 11, no. 4, pp. 407. http://doi.org/10.3390/rs11040407.
http://doi.org/10.3390/rs11040407...
; Shi et al., 2021SHI, H., XIAN, G., AUCH, R., GALLO, K. and ZHOU, Q., 2021. Urban Heat Island and its regional impacts using remotely sensed thermal data: a review of recent developments and methodology. Land, vol. 10, no. 8, pp. 867. http://doi.org/10.3390/land10080867.
http://doi.org/10.3390/land10080867...
; Nguyen et al., 2022NGUYEN, C.T., CHIDTHAISONG, A., LIMSAKUL, A., VARNAKOVIDA, P., EKKAWATPANIT, C., DIEM, P.K. and DIEP, N.T.H., 2022. How do disparate urbanization and climate change imprint on urban thermal variations? A comparison between two dynamic cities in Southeast Asia. Sustainable Cities and Society, vol. 82, pp. 103882. http://doi.org/10.1016/j.scs.2022.103882.
http://doi.org/10.1016/j.scs.2022.103882...
; Mokarram et al., 2023MOKARRAM, M., TARIPANAH, F. and PHAM, T.M., 2023. Investigating the effect of surface urban heat islands on the trend of temperature changes. Advances in Space Research, vol. 72, no. 8, pp. 3150-3169. http://doi.org/10.1016/j.asr.2023.06.048.
http://doi.org/10.1016/j.asr.2023.06.048...
). The Human Modification Index (HMI), based on comprehensive worldwide data from 2016, incorporates 13 human-caused stressors and their anticipated consequences to assess the combined impact of human activities. The stressors were classified into five primary categories: human settlements, transportation, agriculture, electrical infrastructure, and mining and energy generation (Theobald et al., 2020THEOBALD, D.M., KENNEDY, C., CHEN, B., OAKLEAF, J., BARUCH-MORDO, S. and KIESECKER, J., 2020. Earth transformed: detailed mapping of global human modification from 1990 to 2017. Earth System Science Data, vol. 12, no. 3, pp. 1953-1972. http://doi.org/10.5194/essd-12-1953-2020.
http://doi.org/10.5194/essd-12-1953-2020...
). So far, there has been no research conducted in Islamabad to assess the correlation between the HMI, LULC, and LST. This study examined the spatial and temporal correlation between HMI and LST changes in Islamabad, Pakistan. The analysis utilized HMI and LST datasets obtained from Landsat 5-TM and Landsat 8-OLI. The USGS website, accessible at https://earthexplorer.usgs.gov, offers Landsat data spanning the years 1988 to 2016. On the other hand, Earth Engine, available at https://developers.google.com/earth-engine/datasets, gave the HMI number groups. The studied area is the primary destination for migration and tourism in Islamabad Capital Territory. In recent decades, a range of human activities have accelerated the process of urbanization. The spatiotemporal relationship between human activities can be effectively studied in Islamabad Capital Territory due to its rapid urbanization and substantial population influx from neighboring provinces.
2. Materials and Methods
2.1. Study area
The Islamabad Capital Territory (Figure 1) is situated at 33°41’35”N and 73°03’50”E, at an elevation of 540 m, in the Punjab region of north-central Pakistan. It is the 9th biggest city in Pakistan, with a population of 1.12 million, rising from 0.3 to 1.12 million between 1990 and 2020 (PBS, 2017PAKISTAN BUREAU OF STATISTICS – PBS, 2017 [viewed 20 January 2023]. District wise census results [online]. Available from: http://www.pbscensus.gov.pk/sites/default/files/bwpsr/kp/ABBOTTABAD_SUMMARY.pdf
http://www.pbscensus.gov.pk/sites/defaul...
), and has a land area of 906 km2 (Kazmi et al., 2016KAZMI, D.H., LI, J., RUAN, C., ZHAO, S. and LI, Y., 2016. A statistical downscaling model for summer rainfall over Pakistan. Climate Dynamics, vol. 47, no. 7-8, pp. 2653-2666. http://doi.org/10.1007/s00382-016-2990-1.
http://doi.org/10.1007/s00382-016-2990-1...
). Using the Köppen and Geiger classification system, the region's climate is categorized as Cfa (Humid subtropical climate) (Kazmi et al., 2016KAZMI, D.H., LI, J., RUAN, C., ZHAO, S. and LI, Y., 2016. A statistical downscaling model for summer rainfall over Pakistan. Climate Dynamics, vol. 47, no. 7-8, pp. 2653-2666. http://doi.org/10.1007/s00382-016-2990-1.
http://doi.org/10.1007/s00382-016-2990-1...
). The average annual air temperature is about 21.3 °C, while the average annual precipitation is 1291.7 mm. June is the hottest month, while January is the coolest month, with 31.2 °C and 10.1 °C, respectively (Kazmi et al., 2016KAZMI, D.H., LI, J., RUAN, C., ZHAO, S. and LI, Y., 2016. A statistical downscaling model for summer rainfall over Pakistan. Climate Dynamics, vol. 47, no. 7-8, pp. 2653-2666. http://doi.org/10.1007/s00382-016-2990-1.
http://doi.org/10.1007/s00382-016-2990-1...
). The Islamabad Capital Territory is a popular tourist Centre. It is the main transportation point to Pakistan's main tourist areas in the western Himalayas and Karakoram highway. Islamabad has attracted people from all over Pakistan due to job opportunities and high living standards, making it one of Pakistan's most cosmopolitan and urbanized cities.
Location map of the study area (Islamabad Capital Territory, Pakistan) with digital elevation model and main roads.
2.2. Datasets and data processing
The current study investigated the past patterns of LULC, and LST (1988-2016), as well as spatial and temporal relationships between HMI and LST fluctuations in Islamabad, Pakistan, utilizing HMI and LST data. The Landsat satellite data i.e., Landsat 5 TM (Thematic mapper) and Landsat 8 OLI (Operational Land Imager) were obtained from the US Geological Survey for the years 1988, 2002, and 2016 to assess LULC and LST changes. Since HMI data was only available for 2016, Landsat data for LULC and LST changes were selected for 14-year intervals. To minimize possible effects of seasonal variations, satellite images of the same month (May) have been used for all analyzed years. The month of May was chosen for our study because the weather is usually clear (low cloud cover). Table 1 shows the time (day) of Landsat data for the years 1998, 2002, and 2016. Before LULC classification, Landsat images were subjected to a standard pre-treatment process that included radiometric and atmospheric correction as shown in Figure 2. Landsat data were corrected radiometrically to eliminate the impacts of light, view angle, sensor calibration, and topography, and atmospherically to eliminate the effects of atmospheric scattering (Minařík et al., 2019MINAŘÍK, R., LANGHAMMER, J. and HANUŠ, J., 2019. Radiometric and atmospheric corrections of multispectral μMCA camera for UAV spectroscopy. Remote Sensing, vol. 11, no. 20, pp. 2428. http://doi.org/10.3390/rs11202428.
http://doi.org/10.3390/rs11202428...
). The Landsat data corrections were made in ENVI 5.3 using the Fast Line-of-Sight Atmospheric Analysis of Hypercubes (FLAASH) model. The FLAASH is based on the Envi 5.3 software's Moderate Resolution Atmospheric Transmission (MODTRAN) code. It is used for digital number conversion as well as radiance calibration. After uploading Landsat data, the parameters were set as described in the FLAASH user manual for multispectral imagery (Apache Software Foundation, 2009APACHE SOFTWARE FOUNDATION, 2009 [viewed 20 January 2023]. Atmospheric correction module: QUAC and FLAASH user’s guide. Version 4.7 [online]. Delaware, EUA. Available from: https://www.nv5geospatialsoftware.com/portals/0/pdfs/envi/flaash_module.pdf
https://www.nv5geospatialsoftware.com/po...
). The sun angle was corrected in ArcMap 10.5 software using metadata file parameters and the raster calculator. The main problem with different atmospheric corrections for various sensors is the usage of multiple methods and parameters, making it difficult to assess the precise results (Rumora et al., 2020RUMORA, L., MILER, M. and MEDAK, D., 2020. Impact of various atmospheric corrections on sentinel-2 land cover classification accuracy using machine learning classifiers. ISPRS International Journal of Geo-Information, vol. 9, no. 4, pp. 277. http://doi.org/10.3390/ijgi9040277.
http://doi.org/10.3390/ijgi9040277...
). After preprocessing, the Maximum Likelihood Classification (MLC) technique was used to create LULC maps for 1988, 2002, and 2016. In remote sensing, the MLC classification method is often employed, where a pixel is assigned to a certain class depending on how similar it is to the target class (Ahmed and Ahmed, 2012AHMED, B. and AHMED, R., 2012. Modeling urban land cover growth dynamics using multi-temporal satellite images: a case study of Dhaka, Bangladesh. ISPRS International Journal of Geo-Information, vol. 1, no. 1, pp. 3-31. http://doi.org/10.3390/ijgi1010003.
http://doi.org/10.3390/ijgi1010003...
). The probability of a pixel belonging to a certain LULC class determines the likelihood. The LST maps were obtained from thermal bands of Landsat (5TM and 8OLI) satellite data over the same data period. LST from Landsat 8 was calculated using bands 10 and 11. LST was first derived for both thermal bands, and then the mean LST value was calculated in ArcMap 10.5 software using the cell statistics option. Stray light effects on Landsat 8 TIRS were accounted for using the TIRS-on-TIRS method to obtain the true sensor-reaching radiance (Appendix A
Appendix A
Landsat 8 (TIRS) band 10 and 11 map after stray light and destripping treatment.
). The HMI data was obtained from Google Earth Engine (GEE) for 2016 to assess the impacts of human activities on LULC and LST dynamics. The GEE platform, created by the Nature Conservancy and Conservation Science, offers access to the HMI dataset. At a geographical resolution of 1 km, HMI ultimately offers a cumulative assessment of worldwide human land changes. Using spatially detailed global data for 2016, this data set focuses on modeling the physical characteristics of thirteen anthropogenic stressors and their projected consequences. Depending on the extent of land modification, HMI ranges from 0 to 1, with 0 indicating an absence of human activities and 1 denoting the highest degree of change. Five main environmental energy production classes/steps were considered to calculate the HMI composite index. Stressors—human settlement, transportation, agriculture, mining, electrical infrastructure, and energy production were considered to calculate the HMI composite index.
2.2.1. Derivation of Land Cover Maps for the period 1988-2016
The acquired Landsat 5 TM and Landsat 8 OLI data were classified into five LULC classes using the MLC classification method for 1988, 2002, and 2016. The MLC method was chosen for LULC classification in our study area because it produces more accurate results than other LULC classification methods (Lu and Weng, 2007LU, D. and WENG, Q., 2007. A survey of image classification methods and techniques for improving classification performance. International Journal of Remote Sensing, vol. 28, no. 5, pp. 823-870. http://doi.org/10.1080/01431160600746456.
http://doi.org/10.1080/01431160600746456...
). It also has some drawbacks, such as assigning higher likelihood to pixels that leads to misclassification error, which can be adjusted using the fusion technique. Since we used Landsat 4-5 (TM), which lacks the panchromatic band, we applied the MLC method for LULC classification without the fusion technique. The final ladn cover types surpassed the widely accepted accuracy range of 85% (Table 2). Built-up, vegetation, agriculture, bare soil, and water bodies were used to categorize the LULC of the study area. For each LULC class, 40 training samples were selected using baseline data and additional information from various sources (e.g., Google images and ground survey points). The Confusion matrix method was used to evaluate the precision of LULC classification. For the Confusion matrix method, random samples were generated from RGB files for each year, and then accuracy was assessed by placing samples on LULC classified maps using ENVI 5.3 software (Ullah et al., 2019aULLAH, S., AHMAD, K., SAJJAD, R.U., ABBASI, A.M., NAZEER, A. and TAHIR, A.A., 2019a. Analysis and simulation of land cover changes and their impacts on land surface temperature in a lower Himalayan region. Journal of Environmental Management, vol. 245, pp. 348-357. http://doi.org/10.1016/j.jenvman.2019.05.063. PMid:31158687.
http://doi.org/10.1016/j.jenvman.2019.05...
).
2.2.2. Estimation of LST for the period 1988-2016
The thermal data is stored in digital numbers (DN) in Landsat series satellite data. The DN data is converted into LST in the current study by following four major steps recommended by Artis and Carnahan (1982)ARTIS, D.A. and CARNAHAN, W.H., 1982. Survey of emissivity variability in thermography of urban areas. Remote Sensing of Environment, vol. 12, no. 4, pp. 313-329. http://doi.org/10.1016/0034-4257(82)90043-8.
http://doi.org/10.1016/0034-4257(82)9004...
. In a first step, DN was converted into spectral radiance by employing the minimum wavelength (LMIN) and maximum wavelength (LMAX) information from Landsat metadata files (Equation 1). In the second step, the spectral radiance was converted into brightness temperature (BT) using Equation 2. BT was converted from Kelvin to degrees Celsius during the third step using the standard equation for conversion (Equation 3). The final step was the estimation of LST using the BT (°C) by employing Equation 4.
where, LMIN is the minimum wavelength, LMAX is the maximum wavelength, and Lλ is the spectral radiance. For the current study, LMIN and LMAX were 1.238 and 15.600 for Landsat (5) and LMIN = 0.10033 and LMAX = 22.00180 for Landsat 8 (band 10 and 11 both). The K1 = Calibration Constant 1 and K2 = Calibration Constant 2 were obtained from Landsat metadata files. The values of K1 and K2 for two Landsat sensors: K1 = 607.76 and K2 = 1260.56 for Landsat 5; K1 = 774.89 and K2 = 1321.08 for Landsat 8 (band 10); K1 = 480.88 and K2 = 1201.14 for Landsat 8 (band 11). The h (Planck's constant), σ (Boltzmann constant), and c (speed of light) are constants with values of 1.380 649 ∗10−23 J/K, 6. 626 * 10-34J s, and 2.998* 108m/s, respectively. Further, λ and ε are the wavelengths of emitted radiance (the peak response and the average of the limiting wavelengths (λ = 11.5 μm) and surface emissivity. Surface emissivity (ε) was derived from the Proportion of vegetation (PV) which is calculated using NDVI from 1988, 2002, and 2016. The following formulae were used for PV calculation (Equation 5):
After PV calculation surface emissivity was calculated using the following Equation 6 (Sobrino et al., 2004SOBRINO, J.A., JIMÉNEZ-MUÑOZ, J.C. and PAOLINI, L., 2004. Land surface temperature retrieval from LANDSAT TM 5. Remote Sensing of Environment, vol. 90, no. 4, pp. 434-440. http://doi.org/10.1016/j.rse.2004.02.003.
http://doi.org/10.1016/j.rse.2004.02.003...
).
Surface emissivity is used for the adjustment of LST with surface parameters.
2.2.3. Normalization of LST
LST normalization was performed for comparability, as seasonal and topographic variations exist in the LST images taken in different periods. It is inappropriate to directly assess and compare LST from different years and seasons. The key goal of normalization is to bring all variables in proportion. The LST image from 2016 was used as a reference in the current study to normalize the LST images from 1988 and 2002. The LST was normalized by Salama et al. (2012)SALAMA, M.S., VAN DER VELDE, R., ZHONG, L., MA, Y., OFWONO, M. and SU, Z., 2012. Decadal variations of land surface temperature anomalies observed over the Tibetan Plateau by the Special Sensor Microwave Imager (SSM/I) from 1987 to 2008. Climatic Change, vol. 114, no. 3-4, pp. 769-781. http://doi.org/10.1007/s10584-012-0427-3.
http://doi.org/10.1007/s10584-012-0427-3...
by the following formula given in Equation 7:
where,
Normalized LST for the years j (1988 and 2002); LST values of the original image before normalization; =The mean LST for 1988 and 2002; The standard deviation of the LST for 1988 and 2002; The standard deviation of the LST for the reference year i (2016); The mean LST value for the year 2016.
2.2.4. Classification of LST zones
The temperature zones were classed into five categories, i.e., 20 °C to <26 °C, 26 °C to <27 °C, 27 °C to <30 °C, and ≥30 °C (Ullah et al., 2019b)ULLAH, S., TAHIR, A.A., AKBAR, T.A., HASSAN, Q.K., DEWAN, A., KHAN, A.J. and KHAN, M., 2019b. Remote sensing-based quantification of the relationships between land use land cover changes and surface temperature over the Lower Himalayan Region. Sustainability, vol. 11, no. 19, pp. 5492. http://doi.org/10.3390/su11195492.
http://doi.org/10.3390/su11195492...
, to calculate the areas of various LST ranges (Figure 3). This comparison aims to assess trends of different LST ranges from 1988 to 2016.
2.2.5. Correlation between human modification and the LST variations
The relationship between human modification and LST was assessed using Geographically Weighted Regression (GWR) models. The GWR model generated R square values map (Figure 4). For analysis, a grid-based ArcMap format file of HMI and LST was created in ArcMap 10.5 software. The grid file was prepared in three steps: first, a grid was created on the study area shape file, then a combined HMI and LST file was prepared using the “Extract Value to Multiple Point” option in the Spatial Analyst tool, and finally, the combined HMI and LST file was intersected on the study area grid file using “Intersection tool” in ArcMap 10.5 software. The grid-based file consists of values of LST and HMI in each grid. Before grid creation, the HMI file was resampled using the nearest neighborhood resampling method into 30* 30 m resolution equal to Landsat series data. Finally, the GWR method was applied to generate an R square map (Figure 4). The statistical significance was assessed by the R square value.
3. Results and Discussion
3.1. Past pattern of LULC classes changes
The past trends of LULC and LST changes showed two distinct trends (Figure 5): area under built-up class were gradually increasing, whereas vegetation, agriculture, and bare soil areas were decreasing over the study period. The overall accuracy of the MLC classifications for 1988, 2002, and 2016 was 89.15%, 95.08%, and 96.16%, respectively (Table 1 and Table 2).
Between 1988 and 2016, there was an increase of +9.94% in built-up areas due to the conversion of vegetation, bare soil, and agricultural areas into built-up areas (Table 3). The results indicated there was a decrease found in bare soil (-3.81%), agriculture (3.94%), and water bodies (0.18%). This results from the increasing urbanization of the study area during the recent thirty years. Since 1988, rapid population increase, migration from neighboring provinces, especially after the 2005 earthquake, and instability in the eastern and western allied areas have led to urbanization in the studied area. The current study results support the findings of Hokao et al. (2012)HOKAO, K., PHONEKEO, V. and SRIVANIT, M., 2012. Assessing the impact of urbanization on urban thermal environment: a case study of Bangkok Metropolitan. International Journal of Applied, vol. 2, no. 7, pp. 243-256., who documented that social, economic, and political factors caused urban expansion. The rapid urbanization since 1988 is also reflected by a decreasing (-3.81%) trend of vegetation cover (Table 3) . Aslam et al. (2021)ASLAM, A., RANA, I.A. and BHATTI, S.S., 2021. The spatiotemporal of urbanisation and local climate: a case study of Islamabad, Pakistan. Environmental Impact Assessment Review, vol. 91, pp. 106666. http://doi.org/10.1016/j.eiar.2021.106666.
http://doi.org/10.1016/j.eiar.2021.10666...
reported that vegetation in the Islamabad Capital Territory decreased due to rapid expansion and replacing the vegetation cover with impervious surfaces. According to Ullah et al. (2019b)ULLAH, S., TAHIR, A.A., AKBAR, T.A., HASSAN, Q.K., DEWAN, A., KHAN, A.J. and KHAN, M., 2019b. Remote sensing-based quantification of the relationships between land use land cover changes and surface temperature over the Lower Himalayan Region. Sustainability, vol. 11, no. 19, pp. 5492. http://doi.org/10.3390/su11195492.
http://doi.org/10.3390/su11195492...
, massive deforestation and population influx significantly affected LULC dynamics in the past 30 years. Similar findings have been reported by Pham et al. (2015)PHAM, V.C., PHAM, T.T.H., TONG, T.H.A., NGUYEN, T.T.H. and PHAM, N.H., 2015. The conversion of agricultural land in the peri-urban areas of Hanoi (Vietnam): patterns in space and time. Journal of Land Use Science, vol. 10, no. 2, pp. 224-242. http://doi.org/10.1080/1747423X.2014.884643.
http://doi.org/10.1080/1747423X.2014.884...
, who found a negative impact of urbanization on vegetation cover and agricultural land in Vietnam.
3.2. The past pattern of LST Changes for the period 1988-2016
Past patterns of LST changes were obtained using Landsat thermal bands by applying equations explained in section 2, shown in Figure 5. The first three LST classes (low, secondary moderate and moderate) declined from 1988 to 2016 (Figure 6). Over the study period, the region under the low LST class decreased from 1% to 0.06% (Figure 6). Similarly, areas for the secondary moderate and moderate LST classes decreased from 9.44% to 1.79% and 15.32% to 15.02%, respectively. The area within the range of secondary high class also decreased from 61.97% to 34.64%. The region within the range of the high LST class showed an opposite trend and increased from 12.27% to 48.48% (Figure 6). The reasons for the LST variations may be both urbanization and climate change. Rapid urbanization contributed to a significant construction that increased LST, further exacerbated by climate change. Both the warming of cities and global climate change have an impact on the LST of the study area. The majority of LST regions are located in populated areas (Figure 3), followed by vegetation, agricultural, bare soil, and water bodies. These findings support the results of Ahmed and Ahmed (2012)AHMED, B. and AHMED, R., 2012. Modeling urban land cover growth dynamics using multi-temporal satellite images: a case study of Dhaka, Bangladesh. ISPRS International Journal of Geo-Information, vol. 1, no. 1, pp. 3-31. http://doi.org/10.3390/ijgi1010003.
http://doi.org/10.3390/ijgi1010003...
, who found lower LST for flora, agriculture, and water bodies and higher LST for built-up areas and bare soil.
3.3. Average LST variations in different LULC types
We examined the relationship between LST and LULC changes in more detail since LULC changes reducing vegetative cover, increasing LST and generating UHI in urban areas have various negative effects on human health and the ecosystems. Our findings show that the built-up areas, bare soil, and agricultural areas have the greatest mean LST (Figure 7). These results corroborate those made by Al Kafy et al. (2020)KAFY, A.A., RAHMAN, M.S., HASAN, M.M. and ISLAM, M., 2020. Modelling future land use land cover changes and their impacts on land surface temperatures in Rajshahi, Bangladesh. Remote Sensing Applications: Society and Environment, vol. 18, pp. 100314. http://doi.org/10.1016/j.rsase.2020.100314.
http://doi.org/10.1016/j.rsase.2020.1003...
, who evaluated the effect of LULC courses on LST in the Rajshahi area of Bangladesh to examine the relationship between LULC classes and thermal signatures. The current data demonstrate that vegetation has been replaced by built-up areas, increasing the LST of the study region. LST also increased for all classes of LULC and expanded beyond the built-up area (Figure 7). This is in line with Terfa et al. (2020)TERFA, B.K., CHEN, N., ZHANG, X. and NIYOGI, D., 2020. Urbanization in small cities and their significant implications on landscape structures: the case in Ethiopia. Sustainability, vol. 12, no. 3, pp. 1235. http://doi.org/10.3390/su12031235.
http://doi.org/10.3390/su12031235...
, who found that LST increased beyond the built-up area in several cities of Ethiopia. Our results were similar to those of Ren et al. (2008)REN, G., ZHOU, Y., CHU, Z., ZHOU, J., ZHANG, A., GUO, J. and LIU, X., 2008. Urbanization effects on observed surface air temperature trends in North China. Journal of Climate, vol. 21, no. 6, pp. 1333-1348. http://doi.org/10.1175/2007JCLI1348.1.
http://doi.org/10.1175/2007JCLI1348.1...
, in Northern China. However, the LST increase is relatively higher in our study area, possibly due to the rapid urban expansion in the past three decades. The findings of our study suggest the presence of urban warming effects in Islamabad Capital Territory.
3.4. The relationship of HMI with the LST variations
The human modification map for the year 2016 is presented in Figure 8. It showed that the highest human modification occurred in the built-up area, which generally has a high human footprint. The relationship between human modification and LST was evaluated by assessing the relationship between HMI, LULC, and LST as explained in the following sections.
3.4.1. HMI in different temperature zones
The HMI findings for different LST zones revealed that the highest human modification was recorded in the highest LST class, followed by the secondary high, moderate, secondary moderate, and lower LST ranges (Figure 4). The GWR analysis of HMI and LST revealed a substantial spatial relationship, particularly in built-up areas (Figure 4). Our findings were consistent with those published by Chu et al. (2020)CHU, L., OLOO, F., CHEN, B., XIE, M. and BLASCHKE, T., 2020. Assessing the influence of tourism-driven activities on environmental variables on Hainan Island, China. Remote Sensing, vol. 12, no. 17, pp. 2813. http://doi.org/10.3390/rs12172813.
http://doi.org/10.3390/rs12172813...
, who investigated the relationship between HMI and LST on Hainan Island in the South China Sea. High human alterations in higher LST classes indicate that anthropogenic activities resulted in the replacement of vegetation with built-up areas, increasing LST. High human modifications are typically connected with urban expansion, which is generated by a large spontaneous population growth influx of people into cities. Pakistan has experienced substantial urbanization over the last 30 years as a result of a 2% annual population growth rate. It is expected to reach 300 million by 2030 and 450 million by 2050 (PBS, 2017PAKISTAN BUREAU OF STATISTICS – PBS, 2017 [viewed 20 January 2023]. District wise census results [online]. Available from: http://www.pbscensus.gov.pk/sites/default/files/bwpsr/kp/ABBOTTABAD_SUMMARY.pdf
http://www.pbscensus.gov.pk/sites/defaul...
). Political and economic difficulties (Weng, 2001WENG, Q.A., 2001. A remote sensing? GIS evaluation of urban expansion and its impact on surface temperature in the Zhujiang Delta, China. International Journal of Remote Sensing, vol. 22, no. 10, pp. 1999-2014.) and rapid population growth (Aboukorin and Al-Shihri, 2015ABOUKORIN, A. and AL-SHIHRI, F.S., 2015. Rapid urbanization and sustainability in Saudi Arabia: the case of Dammam metropolitan area. Journal of Sustainable Development, vol. 8, no. 9, pp. 52. http://doi.org/10.5539/jsd.v8n9p52.
http://doi.org/10.5539/jsd.v8n9p52...
) are among the primary drivers of urbanization in our research region, and they are not unique contributors to urban expansion
3.4.2. Variation of HMI and mean LST in LULC types
The built-up area has the highest HMI, followed by agricultural land, bare soil, vegetation, and water bodies (Figure 9). The mean LST showed a similar pattern for the study period except for bare soil. The highest mean LST was found in the built-up area, while the lowest was in water bodies. The effect of human activities on LST has been widely reported in the literature (Akbar et al., 2019AKBAR, T.A., HASSAN, Q.K., ISHAQ, S., BATOOL, M., BUTT, H.J. and JABBAR, H., 2019. Investigative spatial distribution and modelling of existing and future urban land changes and its impact on urbanization and economy. Remote Sensing, vol. 11, no. 2, pp. 105. http://doi.org/10.3390/rs11020105.
http://doi.org/10.3390/rs11020105...
; Sajjad and Iqbal, 2012SAJJAD, H. and IQBAL, M., 2012. Impact of urbanization on land use/land cover of Dudhganga watershed of Kashmir Valley, India. International Journal of Urban Sciences, vol. 16, no. 3, pp. 321-339. http://doi.org/10.1080/12265934.2012.743749.
http://doi.org/10.1080/12265934.2012.743...
; Sarmah et al., 2018SARMAH, S., JIA, G., ZHANG, A. and SINGHA, M., 2018. Assessing seasonal trends and variability of vegetation growth from NDVI3g, MODIS NDVI and EVI over South Asia. Remote Sensing Letters, vol. 9, no. 12, pp. 1195-1204. http://doi.org/10.1080/2150704X.2018.1519270.
http://doi.org/10.1080/2150704X.2018.151...
; Yu et al., 2018YU, Z., GUO, X., ZENG, Y., KOGA, M. and VEJRE, H., 2018. Variations in land surface temperature and cooling efficiency of green space in rapid urbanization: the case of Fuzhou city, China. Urban Forestry & Urban Greening, vol. 29, pp. 113-121. http://doi.org/10.1016/j.ufug.2017.11.008.
http://doi.org/10.1016/j.ufug.2017.11.00...
). A study carried out in China (Weng et al., 2004WENG, Q., LU, D. and SCHUBRING, J., 2004. Estimation of land surface temperature–vegetation abundance relationship for urban heat island studies. Remote Sensing of Environment, vol. 89, no. 4, pp. 467-483. http://doi.org/10.1016/j.rse.2003.11.005.
http://doi.org/10.1016/j.rse.2003.11.005...
) found that LST is affected by human activities and increased mean LST in urban impervious surfaces (Iqbal et al., 2012IQBAL, M., RASHID, S.M., SAJJAD, H., SIDDIQUI, M.A. and SIDDIQUI, L., 2012. Anthropogenic impact on landuse/landcover in Dudhganga Watershed of Kashmir Valley, India. International Journal of Geomatics and Geosciences, vol. 2, no. 3, pp. 892-900.; Ahmed and Ahmed, 2012AHMED, B. and AHMED, R., 2012. Modeling urban land cover growth dynamics using multi-temporal satellite images: a case study of Dhaka, Bangladesh. ISPRS International Journal of Geo-Information, vol. 1, no. 1, pp. 3-31. http://doi.org/10.3390/ijgi1010003.
http://doi.org/10.3390/ijgi1010003...
; Reiners et al., 2023REINERS, P., SOBRINO, J. and KUENZER, C., 2023. Satellite-derived land surface temperature dynamics in the context of global change: a review. Remote Sensing, vol. 15, no. 7, pp. 1857. http://doi.org/10.3390/rs15071857.
http://doi.org/10.3390/rs15071857...
). Our results were also consistent with the findings of Isa et al. (2017)ISA, N.A., WAN MOHD, W.M.N. and SALLEH, S.A., 2017. The effects of built-up and green areas on the land surface temperature of Kuala Lumpur City. The International Archives of the Photogrammetry, Remote Sensing and Spatial Information Sciences, vol. 42, no. W5, pp. 107-112. http://doi.org/10.5194/isprs-archives-XLII-4-W5-107-2017.
http://doi.org/10.5194/isprs-archives-XL...
, who found similar results in Kuala Lumpur, Malaysia. It is evident from Figure 9 that those human activities affected LST in all LULC types. High human activities are generally associated with high population density, affecting LST in various parts of the world. There is a very high possibility that human effects might explain UHI and heatwave incidences (Stott et al., 2004STOTT, P.A., STONE, D.A. and ALLEN, M.R., 2004. Human contribution to the European heatwave of 2003. Nature, vol. 432, no. 7017, pp. 610-614. http://doi.org/10.1038/nature03089. PMid:15577907.
http://doi.org/10.1038/nature03089...
).
Map of the R square values between HMI and LST generated by Weighted Regression model in ArcMap 10.5 software.
4. Conclusions
Human activities cause LULC landscape changes, increasing surface radiation levels in urban areas. The study found that LULC classes, such as built-up, increased significantly, whereas agriculture and bare soil decreased respectively. The mean LST results for the various LULC classes indicated that bare soil, agricultural, and vegetation have lower mean LST than built-up areas. It has also been noted that the LST mean has increased across all types of land cover, implying that both climate and urban warming have had an impact on the study region. Human modification dominates in built-up areas, followed by agriculture, bare soil, vegetation, and water bodies (based on LULC data). Similarly, the outcomes of human modification in different LST classes showed that higher LST classes had more human modification than lower LST classes. The present study provides a comprehensive foundation for understanding the relationship between LULC, LST, HMI, and UHI planning and management. Also, this study demonstrates the potential contribution towards the development of sustainable management plans and policies for urban development (i.e. development of policies of urban infrastructure, land utilization and zoning, urban heat stress mitigation strategy) and protection of sensitive ecosystems from human disturbance. The possible limitation of the present study involves an average sample size for LULC classification, therefore, for future research, we recommend larger sample size and high-resolution spot images for LULC classification.
Appendix A Landsat 8 (TIRS) band 10 and 11 map after stray light and destripping treatment.
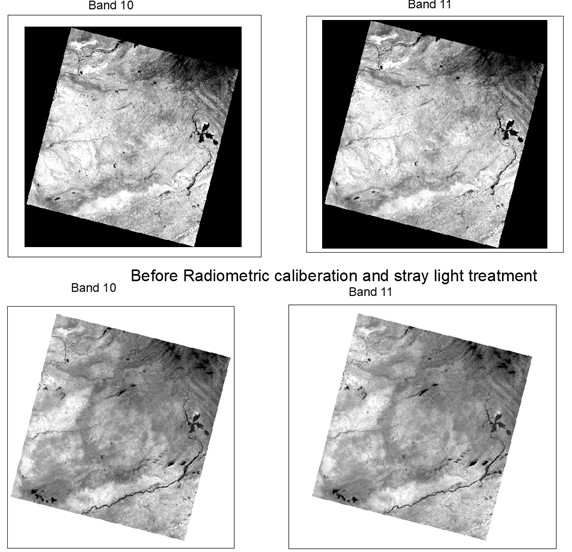
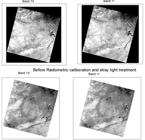
References
- ABOUKORIN, A. and AL-SHIHRI, F.S., 2015. Rapid urbanization and sustainability in Saudi Arabia: the case of Dammam metropolitan area. Journal of Sustainable Development, vol. 8, no. 9, pp. 52. http://doi.org/10.5539/jsd.v8n9p52
» http://doi.org/10.5539/jsd.v8n9p52 - AHMED, B. and AHMED, R., 2012. Modeling urban land cover growth dynamics using multi-temporal satellite images: a case study of Dhaka, Bangladesh. ISPRS International Journal of Geo-Information, vol. 1, no. 1, pp. 3-31. http://doi.org/10.3390/ijgi1010003
» http://doi.org/10.3390/ijgi1010003 - AKBAR, T.A., HASSAN, Q.K., ISHAQ, S., BATOOL, M., BUTT, H.J. and JABBAR, H., 2019. Investigative spatial distribution and modelling of existing and future urban land changes and its impact on urbanization and economy. Remote Sensing, vol. 11, no. 2, pp. 105. http://doi.org/10.3390/rs11020105
» http://doi.org/10.3390/rs11020105 - ALI, K., AHMAD, H., KHAN, N. and JURY, S., 2014. Future of Abies pindrow in Swat District, Northern Pakistan. Journal of Forestry Research, vol. 25, no. 1, pp. 211-214. http://doi.org/10.1007/s11676-014-0446-1
» http://doi.org/10.1007/s11676-014-0446-1 - APACHE SOFTWARE FOUNDATION, 2009 [viewed 20 January 2023]. Atmospheric correction module: QUAC and FLAASH user’s guide. Version 4.7 [online]. Delaware, EUA. Available from: https://www.nv5geospatialsoftware.com/portals/0/pdfs/envi/flaash_module.pdf
» https://www.nv5geospatialsoftware.com/portals/0/pdfs/envi/flaash_module.pdf - ARTIS, D.A. and CARNAHAN, W.H., 1982. Survey of emissivity variability in thermography of urban areas. Remote Sensing of Environment, vol. 12, no. 4, pp. 313-329. http://doi.org/10.1016/0034-4257(82)90043-8
» http://doi.org/10.1016/0034-4257(82)90043-8 - ASLAM, A., RANA, I.A. and BHATTI, S.S., 2021. The spatiotemporal of urbanisation and local climate: a case study of Islamabad, Pakistan. Environmental Impact Assessment Review, vol. 91, pp. 106666. http://doi.org/10.1016/j.eiar.2021.106666
» http://doi.org/10.1016/j.eiar.2021.106666 - BUYANTUYEV, A. and WU, J., 2010. Urban heat islands and landscape heterogeneity: linking spatiotemporal variations in surface temperatures to land-cover and socioeconomic patterns. Landscape Ecology, vol. 25, no. 1, pp. 17-33. http://doi.org/10.1007/s10980-009-9402-4
» http://doi.org/10.1007/s10980-009-9402-4 - CHU, L., OLOO, F., CHEN, B., XIE, M. and BLASCHKE, T., 2020. Assessing the influence of tourism-driven activities on environmental variables on Hainan Island, China. Remote Sensing, vol. 12, no. 17, pp. 2813. http://doi.org/10.3390/rs12172813
» http://doi.org/10.3390/rs12172813 - CONNORS, J.P., GALLETTI, C.S. and CHOW, W.T.L., 2013. Landscape configuration and urban heat island effects: assessing the relationship between landscape characteristics and land surface temperature in Phoenix, Arizona. Landscape Ecology, vol. 28, no. 2, pp. 271-283. http://doi.org/10.1007/s10980-012-9833-1
» http://doi.org/10.1007/s10980-012-9833-1 - COOPS, N.C., WULDER, M.A. and WHITE, J.C., 2006. Identifying and describing forest disturbance and spatial pattern. In: M.A. WULDER and S.E. FRANKLIN, eds. Understanding forest disturbance and spatial pattern. Boca Raton: CRC Press, pp. 31-61.
- DAS, S. and ANGADI, D.P., 2020. Land use-land cover (LULC) transformation and its relation with land surface temperature changes: a case study of Barrackpore Subdivision, West Bengal, India. Remote Sensing Applications: Society and Environment, vol. 19, pp. 100322. http://doi.org/10.1016/j.rsase.2020.100322
» http://doi.org/10.1016/j.rsase.2020.100322 - DAS, T., JANA, A., MANDAL, B. and SUTRADHAR, A., 2022. Spatio-temporal pattern of land use and land cover and its effects on land surface temperature using remote sensing and GIS techniques: a case study of Bhubaneswar city, Eastern India (1991-2021). GeoJournal, vol. 87, suppl. 4, pp. 765-795. http://doi.org/10.1007/s10708-021-10541-z
» http://doi.org/10.1007/s10708-021-10541-z - FU, C., 2003. Potential impacts of human-induced land cover change on East Asia monsoon. Global and Planetary Change, vol. 37, no. 4, pp. 219-229. http://doi.org/10.1016/S0921-8181(02)00207-2
» http://doi.org/10.1016/S0921-8181(02)00207-2 - FU, P. and WENG, Q.A., 2016. time series analysis of urbanization induced land use and land cover change and its impact on land surface temperature with Landsat imagery. Remote Sensing of Environment, vol. 175, pp. 205-214. http://doi.org/10.1016/j.rse.2015.12.040
» http://doi.org/10.1016/j.rse.2015.12.040 - GHOSH, S., KUMAR, D. and KUMARI, R., 2022. Assessing spatiotemporal dynamics of land surface temperature and satellite-derived indices for new town development and suburbanization planning. Urban Governance, vol. 2, no. 1, pp. 144-156. http://doi.org/10.1016/j.ugj.2022.05.001
» http://doi.org/10.1016/j.ugj.2022.05.001 - GUHA, S., GOVIL, H., DEY, A. and GILL, N., 2018. Analytical study of land surface temperature with NDVI and NDBI using Landsat 8 OLI and TIRS data in Florence and Naples city, Italy. European Journal of Remote Sensing, vol. 51, no. 1, pp. 667-678. http://doi.org/10.1080/22797254.2018.1474494
» http://doi.org/10.1080/22797254.2018.1474494 - HERBECK, L.S., UNGER, D., KRUMME, U., LIU, S.M. and JENNERJAHN, T.C., 2011. Typhoon-induced precipitation impact on nutrient and suspended matter dynamics of a tropical estuary affected by human activities in Hainan, China. Estuarine, Coastal and Shelf Science, vol. 93, no. 4, pp. 375-388. http://doi.org/10.1016/j.ecss.2011.05.004
» http://doi.org/10.1016/j.ecss.2011.05.004 - HOKAO, K., PHONEKEO, V. and SRIVANIT, M., 2012. Assessing the impact of urbanization on urban thermal environment: a case study of Bangkok Metropolitan. International Journal of Applied, vol. 2, no. 7, pp. 243-256.
- HU, S., FU, Z., JACKSON SAMUEL, R.D. and ANANDHAN, P., 2021. Application of active remote sensing in confirmation rights and identification of mortgage supply-demand subjects of rural land in Guangdong Province. European Journal of Remote Sensing, vol. 54, no. 2, pp. 396-404. http://doi.org/10.1080/22797254.2020.1790996
» http://doi.org/10.1080/22797254.2020.1790996 - HUANG, G., ZHOU, W. and CADENASSO, M.L., 2011. Is everyone hot in the city? Spatial pattern of land surface temperatures, land cover and neighborhood socioeconomic characteristics in Baltimore, MD. Journal of Environmental Management, vol. 92, no. 7, pp. 1753-1759. http://doi.org/10.1016/j.jenvman.2011.02.006 PMid:21371807.
» http://doi.org/10.1016/j.jenvman.2011.02.006 - IQBAL, M., RASHID, S.M., SAJJAD, H., SIDDIQUI, M.A. and SIDDIQUI, L., 2012. Anthropogenic impact on landuse/landcover in Dudhganga Watershed of Kashmir Valley, India. International Journal of Geomatics and Geosciences, vol. 2, no. 3, pp. 892-900.
- ISA, N.A., WAN MOHD, W.M.N. and SALLEH, S.A., 2017. The effects of built-up and green areas on the land surface temperature of Kuala Lumpur City. The International Archives of the Photogrammetry, Remote Sensing and Spatial Information Sciences, vol. 42, no. W5, pp. 107-112. http://doi.org/10.5194/isprs-archives-XLII-4-W5-107-2017
» http://doi.org/10.5194/isprs-archives-XLII-4-W5-107-2017 - JENERETTE, G.D., HARLAN, S.L., BRAZEL, A., JONES, N., LARSEN, L. and STEFANOV, W.L., 2007. Regional relationships between surface temperature, vegetation, and human settlement in a rapidly urbanizing ecosystem. Landscape Ecology, vol. 22, no. 3, pp. 353-365. http://doi.org/10.1007/s10980-006-9032-z
» http://doi.org/10.1007/s10980-006-9032-z - KAFY, A.A., RAHMAN, M.S., HASAN, M.M. and ISLAM, M., 2020. Modelling future land use land cover changes and their impacts on land surface temperatures in Rajshahi, Bangladesh. Remote Sensing Applications: Society and Environment, vol. 18, pp. 100314. http://doi.org/10.1016/j.rsase.2020.100314
» http://doi.org/10.1016/j.rsase.2020.100314 - KAR, S.K. and LIOU, Y.A., 2019. Influence of land use and land cover change on the formation of local lightning. Remote Sensing, vol. 11, no. 4, pp. 407. http://doi.org/10.3390/rs11040407
» http://doi.org/10.3390/rs11040407 - KAZMI, D.H., LI, J., RUAN, C., ZHAO, S. and LI, Y., 2016. A statistical downscaling model for summer rainfall over Pakistan. Climate Dynamics, vol. 47, no. 7-8, pp. 2653-2666. http://doi.org/10.1007/s00382-016-2990-1
» http://doi.org/10.1007/s00382-016-2990-1 - LU, D. and WENG, Q., 2007. A survey of image classification methods and techniques for improving classification performance. International Journal of Remote Sensing, vol. 28, no. 5, pp. 823-870. http://doi.org/10.1080/01431160600746456
» http://doi.org/10.1080/01431160600746456 - MINAŘÍK, R., LANGHAMMER, J. and HANUŠ, J., 2019. Radiometric and atmospheric corrections of multispectral μMCA camera for UAV spectroscopy. Remote Sensing, vol. 11, no. 20, pp. 2428. http://doi.org/10.3390/rs11202428
» http://doi.org/10.3390/rs11202428 - MOKARRAM, M., TARIPANAH, F. and PHAM, T.M., 2023. Investigating the effect of surface urban heat islands on the trend of temperature changes. Advances in Space Research, vol. 72, no. 8, pp. 3150-3169. http://doi.org/10.1016/j.asr.2023.06.048
» http://doi.org/10.1016/j.asr.2023.06.048 - NGUYEN, C.T., CHIDTHAISONG, A., LIMSAKUL, A., VARNAKOVIDA, P., EKKAWATPANIT, C., DIEM, P.K. and DIEP, N.T.H., 2022. How do disparate urbanization and climate change imprint on urban thermal variations? A comparison between two dynamic cities in Southeast Asia. Sustainable Cities and Society, vol. 82, pp. 103882. http://doi.org/10.1016/j.scs.2022.103882
» http://doi.org/10.1016/j.scs.2022.103882 - NURUZZAMAN, M., 2015. Urban heat island: causes, effects and mitigation measures-a review. International Journal of Environmental Monitoring and Analysis, vol. 3, no. 2, pp. 67-73. http://doi.org/10.11648/j.ijema.20150302.15
» http://doi.org/10.11648/j.ijema.20150302.15 - PAKISTAN BUREAU OF STATISTICS – PBS, 2017 [viewed 20 January 2023]. District wise census results [online]. Available from: http://www.pbscensus.gov.pk/sites/default/files/bwpsr/kp/ABBOTTABAD_SUMMARY.pdf
» http://www.pbscensus.gov.pk/sites/default/files/bwpsr/kp/ABBOTTABAD_SUMMARY.pdf - PHAM, V.C., PHAM, T.T.H., TONG, T.H.A., NGUYEN, T.T.H. and PHAM, N.H., 2015. The conversion of agricultural land in the peri-urban areas of Hanoi (Vietnam): patterns in space and time. Journal of Land Use Science, vol. 10, no. 2, pp. 224-242. http://doi.org/10.1080/1747423X.2014.884643
» http://doi.org/10.1080/1747423X.2014.884643 - RAHIMI, L., 2019 [viewed 20 January 2023]. The relationship between the use of land cover and urban thermal islands using Landsat 8: case study of Sanandaj. Journal of Radar and Optical Remote Sensing [online], vol. 2, no. 4, pp. 79-92. Available from: https://sanad.iau.ir/journal/jrors/article_669740.html
» https://sanad.iau.ir/journal/jrors/article_669740.html - REINERS, P., SOBRINO, J. and KUENZER, C., 2023. Satellite-derived land surface temperature dynamics in the context of global change: a review. Remote Sensing, vol. 15, no. 7, pp. 1857. http://doi.org/10.3390/rs15071857
» http://doi.org/10.3390/rs15071857 - REN, G., ZHOU, Y., CHU, Z., ZHOU, J., ZHANG, A., GUO, J. and LIU, X., 2008. Urbanization effects on observed surface air temperature trends in North China. Journal of Climate, vol. 21, no. 6, pp. 1333-1348. http://doi.org/10.1175/2007JCLI1348.1
» http://doi.org/10.1175/2007JCLI1348.1 - RUMORA, L., MILER, M. and MEDAK, D., 2020. Impact of various atmospheric corrections on sentinel-2 land cover classification accuracy using machine learning classifiers. ISPRS International Journal of Geo-Information, vol. 9, no. 4, pp. 277. http://doi.org/10.3390/ijgi9040277
» http://doi.org/10.3390/ijgi9040277 - SAHANI, J., KUMAR, P., DEBELE, S. and EMMANUEL, R., 2022. Heat risk of mortality in two different regions of the United Kingdom. Sustainable Cities and Society, vol. 80, pp. 103758. http://doi.org/10.1016/j.scs.2022.103758
» http://doi.org/10.1016/j.scs.2022.103758 - SAJJAD, H. and IQBAL, M., 2012. Impact of urbanization on land use/land cover of Dudhganga watershed of Kashmir Valley, India. International Journal of Urban Sciences, vol. 16, no. 3, pp. 321-339. http://doi.org/10.1080/12265934.2012.743749
» http://doi.org/10.1080/12265934.2012.743749 - SALAMA, M.S., VAN DER VELDE, R., ZHONG, L., MA, Y., OFWONO, M. and SU, Z., 2012. Decadal variations of land surface temperature anomalies observed over the Tibetan Plateau by the Special Sensor Microwave Imager (SSM/I) from 1987 to 2008. Climatic Change, vol. 114, no. 3-4, pp. 769-781. http://doi.org/10.1007/s10584-012-0427-3
» http://doi.org/10.1007/s10584-012-0427-3 - SARMAH, S., JIA, G., ZHANG, A. and SINGHA, M., 2018. Assessing seasonal trends and variability of vegetation growth from NDVI3g, MODIS NDVI and EVI over South Asia. Remote Sensing Letters, vol. 9, no. 12, pp. 1195-1204. http://doi.org/10.1080/2150704X.2018.1519270
» http://doi.org/10.1080/2150704X.2018.1519270 - SHI, H., XIAN, G., AUCH, R., GALLO, K. and ZHOU, Q., 2021. Urban Heat Island and its regional impacts using remotely sensed thermal data: a review of recent developments and methodology. Land, vol. 10, no. 8, pp. 867. http://doi.org/10.3390/land10080867
» http://doi.org/10.3390/land10080867 - SOBRINO, J.A., JIMÉNEZ-MUÑOZ, J.C. and PAOLINI, L., 2004. Land surface temperature retrieval from LANDSAT TM 5. Remote Sensing of Environment, vol. 90, no. 4, pp. 434-440. http://doi.org/10.1016/j.rse.2004.02.003
» http://doi.org/10.1016/j.rse.2004.02.003 - STOTT, P.A., STONE, D.A. and ALLEN, M.R., 2004. Human contribution to the European heatwave of 2003. Nature, vol. 432, no. 7017, pp. 610-614. http://doi.org/10.1038/nature03089 PMid:15577907.
» http://doi.org/10.1038/nature03089 - TERFA, B.K., CHEN, N., ZHANG, X. and NIYOGI, D., 2020. Urbanization in small cities and their significant implications on landscape structures: the case in Ethiopia. Sustainability, vol. 12, no. 3, pp. 1235. http://doi.org/10.3390/su12031235
» http://doi.org/10.3390/su12031235 - THEOBALD, D.M., KENNEDY, C., CHEN, B., OAKLEAF, J., BARUCH-MORDO, S. and KIESECKER, J., 2020. Earth transformed: detailed mapping of global human modification from 1990 to 2017. Earth System Science Data, vol. 12, no. 3, pp. 1953-1972. http://doi.org/10.5194/essd-12-1953-2020
» http://doi.org/10.5194/essd-12-1953-2020 - ULLAH, S., AHMAD, K., SAJJAD, R.U., ABBASI, A.M., NAZEER, A. and TAHIR, A.A., 2019a. Analysis and simulation of land cover changes and their impacts on land surface temperature in a lower Himalayan region. Journal of Environmental Management, vol. 245, pp. 348-357. http://doi.org/10.1016/j.jenvman.2019.05.063 PMid:31158687.
» http://doi.org/10.1016/j.jenvman.2019.05.063 - ULLAH, S., TAHIR, A.A., AKBAR, T.A., HASSAN, Q.K., DEWAN, A., KHAN, A.J. and KHAN, M., 2019b. Remote sensing-based quantification of the relationships between land use land cover changes and surface temperature over the Lower Himalayan Region. Sustainability, vol. 11, no. 19, pp. 5492. http://doi.org/10.3390/su11195492
» http://doi.org/10.3390/su11195492 - ULLAH, W., AHMAD, K., ULLAH, S., TAHIR, A.A., JAVED, M.F., NAZIR, A., NAZIR, A., ABBASI, A.M., AZIZ, M. and MOHAMED, A., 2023. Analysis of the relationship among land surface temperature (LST), land use land cover (LULC), and normalized difference vegetation index (NDVI) with topographic elements in the lower Himalayan region. Heliyon, vol. 9, no. 2, e13322. http://doi.org/10.1016/j.heliyon.2023.e13322 PMid:36825192.
» http://doi.org/10.1016/j.heliyon.2023.e13322 - VAIDYANATHAN, A., MALILAY, J., SCHRAMM, P. and SAHA, S., 2020. MMWR - heat-related deaths: United States, 2004-2018. MMWR. Morbidity and Mortality Weekly Report, vol. 69, no. 24, pp. 729-734. http://doi.org/10.15585/mmwr.mm6924a1 PMid:32555133.
» http://doi.org/10.15585/mmwr.mm6924a1 - VERBURG, P.H. and OVERMARS, K.P., 2009. Combining top-down and bottom-up dynamics in land use modeling: exploring the future of abandoned farmlands in Europe with the Dyna-CLUE model. Landscape Ecology, vol. 24, no. 9, pp. 1167-1181. http://doi.org/10.1007/s10980-009-9355-7
» http://doi.org/10.1007/s10980-009-9355-7 - WENG, Q., LU, D. and SCHUBRING, J., 2004. Estimation of land surface temperature–vegetation abundance relationship for urban heat island studies. Remote Sensing of Environment, vol. 89, no. 4, pp. 467-483. http://doi.org/10.1016/j.rse.2003.11.005
» http://doi.org/10.1016/j.rse.2003.11.005 - WENG, Q.A., 2001. A remote sensing? GIS evaluation of urban expansion and its impact on surface temperature in the Zhujiang Delta, China. International Journal of Remote Sensing, vol. 22, no. 10, pp. 1999-2014.
- WILLIE, Y.A., PILLAY, R., ZHOU, L. and ORIMOLOYE, I.R., 2019. Orimoloye, I.R. Monitoring spatial pattern of land surface thermal characteristics and urban growth: a case study of King Williams using remote sensing and GIS. Earth Science Informatics, vol. 12, no. 4, pp. 447-464. http://doi.org/10.1007/s12145-019-00391-2
» http://doi.org/10.1007/s12145-019-00391-2 - XIUWAN, C., 2002. Using remote sensing and GIS to analyse land cover change and its impacts on regional sustainable development. International Journal of Remote Sensing, vol. 23, no. 1, pp. 107-124. http://doi.org/10.1080/01431160010007051
» http://doi.org/10.1080/01431160010007051 - YAMAMOTO, Y., 2005. Measures to mitigate urban heat islands. Quartely Review, vol. 18, pp. 65-83.
- YU, Z., GUO, X., ZENG, Y., KOGA, M. and VEJRE, H., 2018. Variations in land surface temperature and cooling efficiency of green space in rapid urbanization: the case of Fuzhou city, China. Urban Forestry & Urban Greening, vol. 29, pp. 113-121. http://doi.org/10.1016/j.ufug.2017.11.008
» http://doi.org/10.1016/j.ufug.2017.11.008 - ZHANG, Y., BALZTER, H., LIU, B. and CHEN, Y., 2016. Analyzing the impacts of urbanization and seasonal variation on land surface temperature based on subpixel fractional covers using Landsat images. IEEE Journal of Selected Topics in Applied Earth Observations and Remote Sensing, vol. 10, no. 4, pp. 1344-1356. http://doi.org/10.1109/JSTARS.2016.2608390
» http://doi.org/10.1109/JSTARS.2016.2608390 - ZHOU, X. and WANG, Y.C., 2011. Dynamics of land surface temperature in response to land‐use/cover change. Geographical Research, vol. 49, no. 1, pp. 23-36. http://doi.org/10.1111/j.1745-5871.2010.00686.x
» http://doi.org/10.1111/j.1745-5871.2010.00686.x
Publication Dates
-
Publication in this collection
12 Aug 2024 -
Date of issue
2024
History
-
Received
22 Dec 2023 -
Accepted
12 Feb 2024